Lars Lindemann
Seminar Information
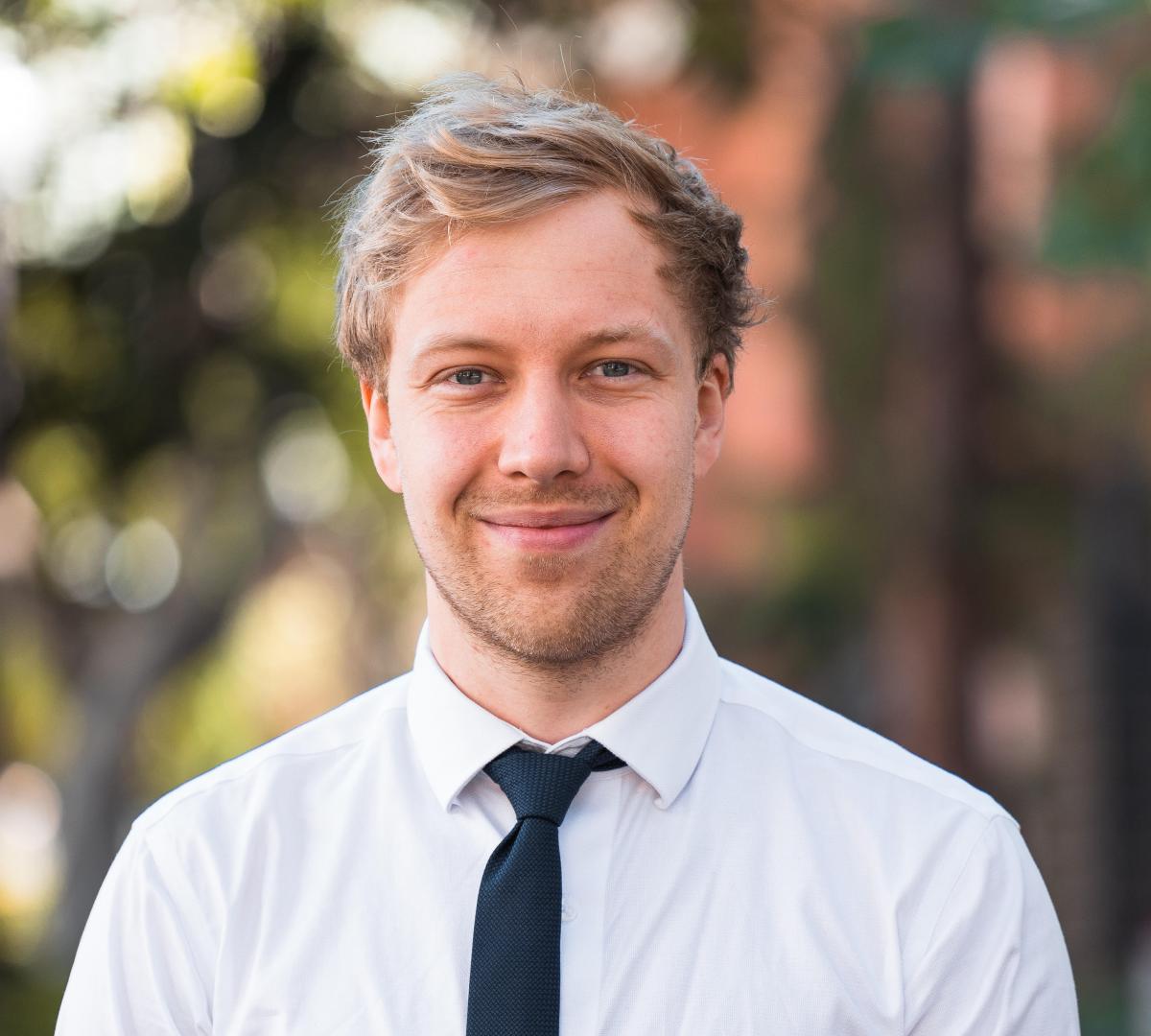
Learning-enabled autonomous systems promise to enable many future technologies such as autonomous driving, intelligent transportation, and robotics. Accelerated by advances in machine learning and AI, there has been tremendous success in the design of learning-enabled autonomous systems. However, these exciting developments are accompanied by new fundamental challenges that arise regarding the safety and reliability of these increasingly complex control systems in which sophisticated algorithms interact with unknown dynamic environments. In this talk, I will provide new insights and discuss exciting opportunities to address these challenges.
I will start by motivating the need for an algorithmic and formal view on systems theory where control algorithms are learning-enabled, formally verified, real-time capable, and reactive to the physical world. Imperfect learning and unknowns in the environment require control techniques to rigorously account for uncertainties. I advocate for the use of conformal prediction (CP) — a statistical tool for uncertainty quantification — due to its simplicity, generality, and efficiency as opposed to existing optimization techniques that are either conservative or not scalable. I first provide an introduction to CP for the non-expert. My goal is then to show how we can use CP to solve the problem of designing probabilistically safe control algorithms in dynamic environments. Specifically, we will design a model predictive controller in conjunction with (i) learning-enabled trajectory predictors to obtain predictions of the environment, and (ii) conformal prediction regions quantifying uncertainty of these predictions. We will also discuss how to deal with high-level temporal logic specifications and distribution shifts that arise when the deployed learning-enabled system changes.
Lars Lindemann is an Assistant Professor in the Thomas Lord Department of Computer Science at the University of Southern California (USC) where he leads the Safe Autonomy and Intelligent Distributed Systems (SAIDS) lab. At USC, he is also the Associate Director of the Center for Autonomy and Artificial Intelligence and member of the Ming Hsieh Department of Electrical and Computer Engineering (by courtesy) and the Robotics and Autonomous Systems Center. Between 2020 and 2022, he was a Postdoctoral Fellow in the Department of Electrical and Systems Engineering at the University of Pennsylvania. Prior to that, he received the Ph.D. degree in Electrical Engineering from KTH Royal Institute of Technology in 2020. His research interests include systems and control theory, formal methods, machine learning, and autonomous systems. Professor Lindemann received the Outstanding Student Paper Award at the 58th IEEE Conference on Decision and Control and the Student Best Paper Award (as an advisor) at the 60th IEEE Conference on Decision and Control. He was finalist for the Best Paper Award (as an advisor) at the 2024 International Conference on Cyber-Physical Systems, the Best Paper Award at the 2022 Conference on Hybrid Systems: Computation and Control, and the Best Student Paper Award at the 2018 American Control Conference.