Hao Zhu
The University of Texas at Austin
Seminar Information
~ Meeting ID: 957 5240 0533
~ Seminar Recording Available: Please contact seminar coordinator, Jake Blair at (j1blair@eng.ucsd.edu)
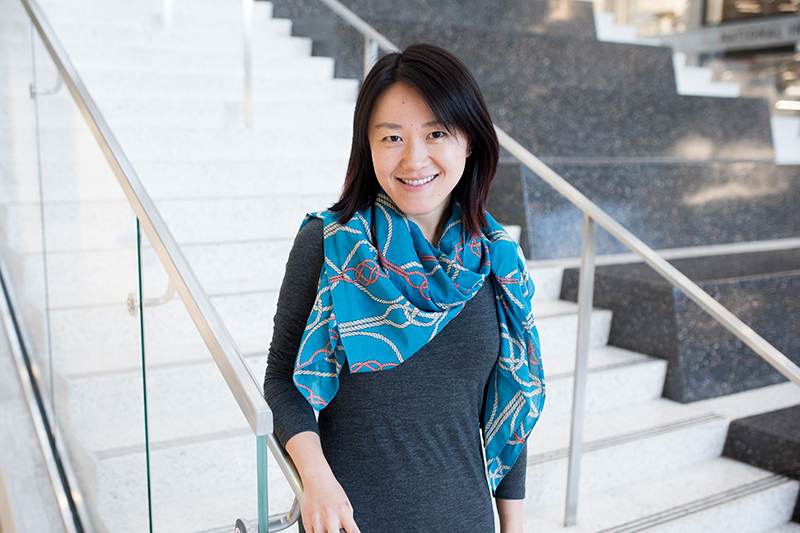
Recent years have witnessed rapid transformations of contemporary advances in machine learning (ML) and data science to aid the transition of energy systems into a truly sustainable, resilient, and distributed infrastructure. A blind application of the latest-and-greatest ML algorithms to solve stylized grid operation problems, however, may fail to recognize the underlying physics models or safety constraint requirements. This talk will introduce two examples of bridging physics-aware and risk-aware ML advances into efficient and reliable grid operations. First, we develop a topology-aware approach using graph neural networks (GNNs) to predict the price and line congestion as the outputs of real-time AC optimal power flow problem. Building upon the underlying relation between prices and topology, this proposed solution significantly reduces the model complexity of existing end-to-end ML methods while efficiently adapting to varying grid topology. Second, we put forth a risk-aware ML method to ensure the safety guarantees of data-driven, scalable reactive power dispatch policies in distribution grids. An adaptive sampling method is leveraged to improve the gradient computation of conditional variance-at-risk (CVaR) and attain guaranteed voltage violation performance. Our proposed algorithms have demonstrated the advantages of incorporating power system modeling into the design of learning-enabled power system operations.
Hao Zhu is currently an Assistant Professor of Electrical and Computer Engineering (ECE) at The University of Texas at Austin. She received the B.S. degree from Tsinghua University in 2006, and the M.Sc. and Ph.D. degrees from the University of Minnesota in 2009 and 2012, all in electrical engineering. From 2012 to 2017, she was a Postdoctoral Research Associate and then an Assistant Professor of ECE at the University of Illinois at Urbana-Champaign. Her research focuses on developing innovative algorithmic solutions for learning and optimization problems in future energy systems. Her current research interests include physics-aware and risk-aware machine learning for power systems, and the design of energy management system under the cyber-physical coupling. Dr. Zhu is a recipient of the NSF CAREER Award (2017), an invited attendee of the US NAE Frontier of Engineering Symposium (2020), as well as the faculty advisor for three Best Student Papers awarded at the North American Power Symposium. She is currently a member of the IEEE Power & Energy Society (PES) Long Range Planning (LRP) Committee and an Associate Editor for the IEEE Transactions on Smart Grid.