Yuhua Zhu
Seminar Information
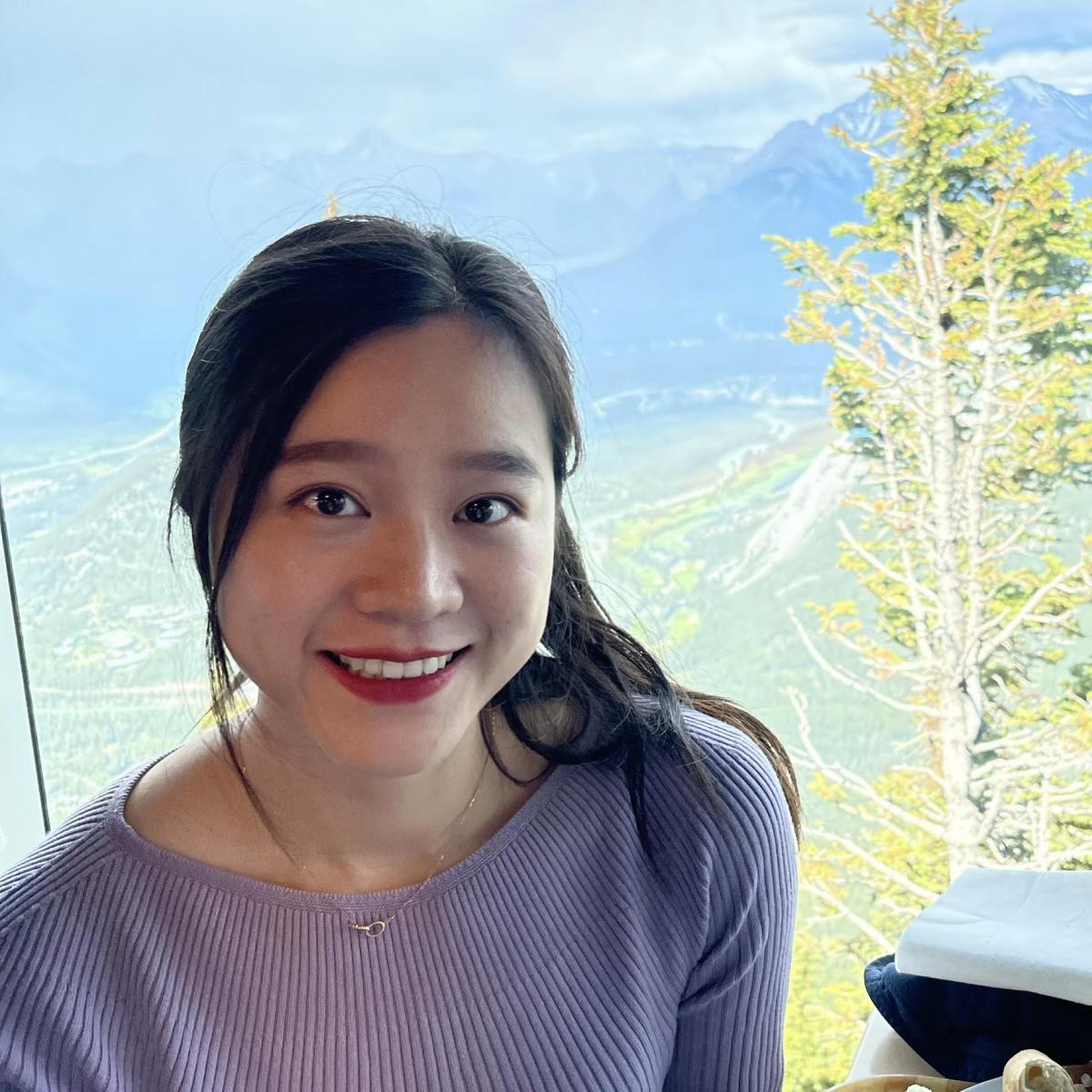
In this talk, I will build the connection between Hamilton-Jacobi-Bellman equations (HJB) and the multi-armed bandit (MAB) problems. HJB is an important equation in solving stochastic optimal control problems. MAB is a widely used paradigm for studying the exploration-exploitation trade-off in sequential decision making under uncertainty. This is the first work that establishes this connection in a general setting. I will present an efficient algorithm for solving MAB problems based on this connection and demonstrate its practical applications. This is a joint work with Lexing Ying and Zach Izzo from Stanford University.
Yuhua Zhu is an assistant professor at UC San Diego, where she holds a joint appointment in the Halicioğlu Data Science Institute (HDSI) and the Department of Mathematics. Previously, she was a Postdoctoral Fellow at Stanford University, and received her Ph.D. from UW-Madison. Her work builds the bridge between differential equations and machine learning, spanning the areas of reinforcement learning, stochastic optimization, sequential decision-making, and uncertainty quantification.