Rudy Geelen
Seminar Information
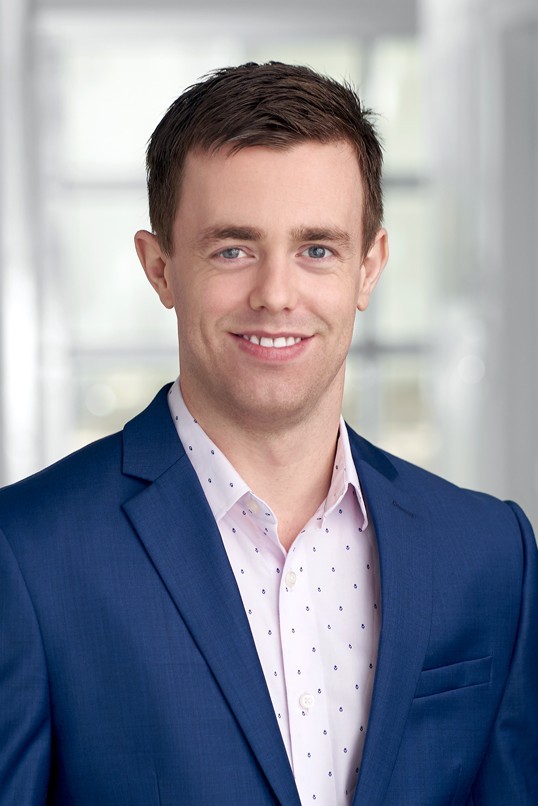
The rapidly increasing demand for computer simulations of complex physical, chemical, and other processes places a significant burden on the shoulders of computational scientists and engineers. Despite the remarkable rise of available computer resources and computing technologies, the need for model order reduction to cope with these problems is an ever-present reality. Reduced-order models are imperative in making computationally tractable outer-loop applications that require simulating systems for many scenarios with different parameters and under varying inputs. They require that one numerically solves the differential equations describing the physical system of interest in low-dimensional reduced spaces, in contrast to the original full-order models. However, traditional model reduction techniques often fail to identify a low-dimensional linear subspace for approximating the solution to many physics-based simulations.
In this talk I will present a novel approach for learning cost-efficient latent space representations in physics-based simulation models by means of nonlinear dimension reduction. Our perspective is driven by the need for low-dimensional and interpretable representations that can be postulated through a simple basis expansion, providing a way to embed physical constraints and preserve important solution features. In particular, we describe a data-driven method for learning nonlinear state approximations of polynomial form via either (i) a proper orthogonal decomposition or (ii) an alternating minimization based approach. Combining such polynomial manifold constructions with the operator inference method for projection-based model reduction leads to a highly scalable, non-intrusive approach for learning reduced-order dynamical-system models. The presented numerical results focus on the initial presentation and demonstration of the methodology by illustrating the gains in efficiency that can be achieved over approximation in linear subspaces.
Rudy Geelen is a postdoctoral researcher working with Karen Willcox at the Oden Institute for Computational Engineering and Sciences. He joined UT Austin as a Peter O'Donnell Postdoctoral Fellow in 2020. Before joining UT Austin, he received a Ph.D. in Mechanical Engineering from Duke University. He holds B.S. and M.S. degrees in Mechanical Engineering from the Eindhoven University of Technology in the Netherlands. He is interested in the broad area of computational science and engineering with a strong focus on computational mechanics, model order reduction and scientific machine learning. As such, his research is interdisciplinary, both with respect to computational-science domains and real-life engineering applications.