Lola Botman
Katholieke Universiteit Leuven
Seminar Information
Engineering Building Unit 2 (EBU2)
Room 479
Seminar Recording Available: Please contact seminar coordinator, Jake Blair at (j1blair@eng.ucsd.edu)
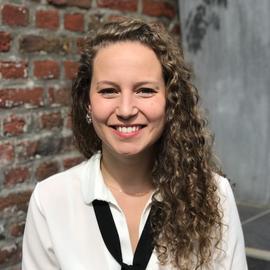
We are in the middle of the energy transition. We consume more electricity than ever before, think of electric vehicles, and heat pumps. Not only that, but we also consume differently, think of renewable energies, such as solar panels on your roof. How is our electric grid, which was built many years ago, supposed to deal with that? In my PhD, I aim to leverage the vast amount of data, recently made available thanks to smart meters to forecast individual households' consumption. These forecasts allow the detection of congestion early on and can serve as a trigger for mitigation strategies.
I first focused on long-term forecasting [1], at the household level, the method I will present consists of three steps: clustering the data using k-means, prediction using an ensemble of forecasts based on the historical median distribution among similar households and smoothing the predictions to remove weather-dependent patterns. The method is highly accurate as it finished third in the IEEE-CIS competition (and ranks first when leveraging insights from another team) and can forecast long-term household consumption with incomplete data.
I then worked on short-term household forecasting. It is even more challenging because individual household profiles are highly stochastic. Even if you know you are going to cook tonight, you don’t know the exact time at which you will start cooking, so how is an algorithm supposed to figure this out? Point forecasting methods cannot capture this efficiently. To have insights into the uncertainty of the prediction, probabilistic methods should be developed. I implemented five methods on three different datasets. Four out of the five methods are individual models, meaning that one model is required per household: (1) Feedforward Neural Network followed by empirical quantiles, (2) Quantile Regression, (3) Quantile Regression Averaging (4) a variation on Quantile Regression Averaging. The Feedforward Neural Network followed by empirical quantiles is a novel method I proposed in this paper [2]. The other methods have been inspired by successful results in other short-term forecasting applications and are used as benchmarking. Finally, I also propose a global approach, meaning that one model is used for all profiles. The approach is based on selecting similar relative consumption profiles using k-nearest neighbors and then averaging using empirical quantiles. The global approach outperforms all other methods on two datasets. It also has the major advantage of requiring only seven days of historical data to make predictions for a given household.
I will share the insights that I have acquired by developing these forecasting algorithms for household consumption data.
[1] L. Botman et al., "A Scalable Ensemble Approach to Forecast the Electricity Consumption of Households," in IEEE Transactions on Smart Grid, vol. 14, no. 1, pp. 757-768, Jan. 2023, doi: 10.1109/TSG.2022.3191399.
[2] L. Botman et al., "A scalable method for probabilistic short-term forecasting of individual households consumption in low voltage grids,"; in Proc. IEEE PES Grid Edge Technologies Conference & Exposition 2023.
As a PhD researcher at the engineering faculty of KU Leuven, I am focusing on times series forecasting in the energy sector. My goal is to develop novel and accurate algorithms, leveraging smart meter data, to enable the energy transition on the low voltage grid level. My PhD is part of the AI Flanders Research program, funded by the Flemish government. More specifically, I work in collaboration with the computer science department of the KU Leuven, VITO -- the Flemish institute for technological research -- and Fluvius -- the main distribution system operator in Belgium.
I previously worked as a consultant at Sopra Steria in digital transformation for 18 months. This first experience in the private sector strengthened communication, problem-solving and client facing skills. I learned to work in a structured way, to deliver under tight deadlines and to be part of a team.
My favorite project was the study we realized to define the Smart City Strategy of the Brussels Region. It involved gathering and meeting all the actors, e.g. Belgium's telecommunication operators, the city council, and mapping all the existing and upcoming projects in IoT, energy, sustainability, and so on. More specifically, I conducted workshops and performed desk research to collect and document the AS IS situation, I collaborated on defining the TO BE target, using a reference architecture framework, and then defined and prioritized the actions to be implemented and their translation into a roadmap.
As a side project, I launched the DiversiTeam: an internal initiative at the BeNeLux (Belgium, Netherlands and Luxembourg) level towards more diversity and inclusion (D&I) within the company. I built a group of interested employees to work on the initiative, defined its scope and mission and led brainstorming sessions to find solutions towards more D&I. I also participated in conferences and seminars on the topic of D&I.
This mixed background in the corporate and academic sector made me realize that a gap needs to be bridged between the knowledge and the culture in research and industry, in both directions, and I wish to be a part of that.