Changliu Liu
Seminar Information
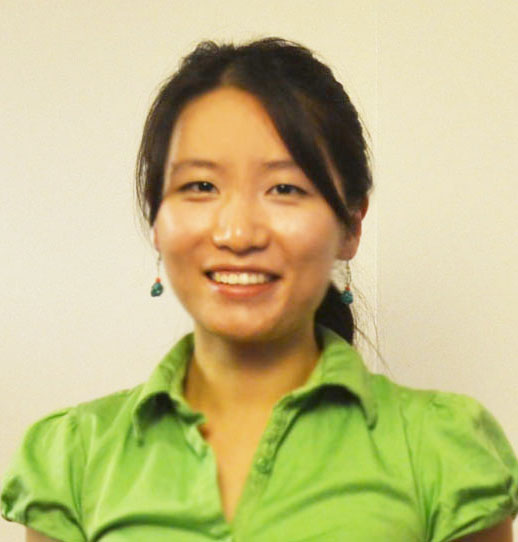
This talk will cover some of our recent work that enables provable safe control of robots when there are dynamic uncertainties and constraints. The safe control is designed under the framework of energy-function-based methods, where a scalar energy function (also known as barrier function, safety index, etc) needs to be synthesized first such that safe states are with low energy, then the safety controller will be synthesized to dissipate the system energy. When there are uncertainties and constraints, it is challenging to obtain a qualified energy function to ensure that there always exists a feasible control to dissipate the energy and will result in forward invariance in the safe set. This talk will introduce several approaches to synthesize such a qualified energy function, including an analytical approach, an evolutionary optimization approach, a sum-of-square approach, and adversarial optimization approaches. Then we will conclude with applications of safe control to enable trustworthy human robot interactions.
Dr. Changliu Liu is an assistant professor in the Robotics Institute, School of Computer Science, Carnegie Mellon University (CMU), where she leads the Intelligent Control Lab. Prior to joining CMU, Dr. Liu was a postdoc at Stanford Intelligent Systems Laboratory. She received her Ph.D. from University of California at Berkeley and her dual bachelor degrees from Tsinghua University. Her research interests lie in the design and verification of intelligent systems with applications to manufacturing and transportation. She published the book “Designing robot behavior in human-robot interactions” with CRC Press in 2019. She initiated and has been organizing the international verification of neural network competition (VNN-COMP) since 2020. Her work is recognized by NSF Career Award, Amazon Research Award, and Ford URP Award.